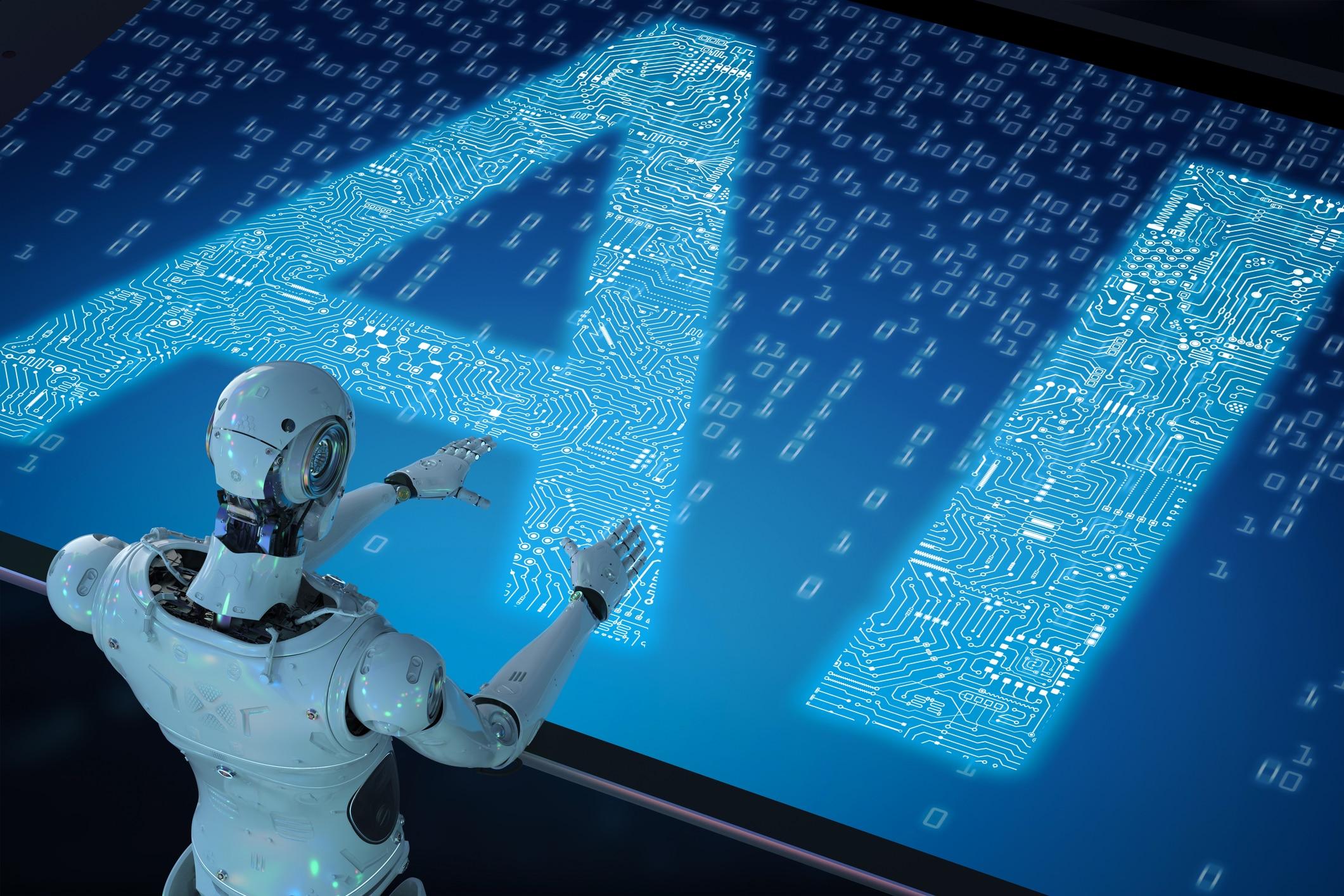
AI for predictive analytics
In a world awash with data, the ability to discern patterns and anticipate future trends has become paramount. Enter artificial intelligence—a potent ally in the realm of predictive analytics. Beyond just numbers and algorithms, AI represents a transformative lens through which businesses can glean invaluable insights, make informed decisions, and stay one step ahead of the competition. This article delves into the capabilities of AI in shaping predictive analytics, exploring its techniques, applications across industries, and the essential role it plays in navigating an increasingly complex landscape. Whether you’re a seasoned data professional or a curious newcomer, understanding the synergy between AI and predictive analytics is not just relevant; it’s essential for thriving in an age defined by rapid change and innovation. Join us as we unravel the intricacies of this dynamic duo and discover how they can unlock new possibilities for informed decision-making.
Table of Contents
- Embracing Predictive Insights Through AI Technology
- Unveiling the Mechanics of AI-Driven Analytics
- Enhancing Decision-Making with Predictive Models
- Best Practices for Implementing AI in Predictive Strategies
- Concluding Remarks
Embracing Predictive Insights Through AI Technology
As organizations strive to stay ahead of the curve, has become imperative. AI-driven predictive analytics transforms raw data into actionable forecasts, enabling companies to anticipate market trends, optimize operations, and enhance decision-making processes. This shift not only minimizes risk but also identifies new opportunities, reshaping the traditional approach to strategic planning. The integration of AI allows for a multi-faceted analysis, leveraging historical data and real-time input to provide a comprehensive view of potential future scenarios.
Key benefits of implementing predictive insights with AI include:
- Enhanced Accuracy: Machine learning algorithms continuously improve their predictive capabilities, leading to increasingly precise forecasts.
- Resource Optimization: Businesses can allocate resources more effectively by understanding demand patterns and resource needs.
- Strategic Decision-Making: With AI insights, organizations can make informed choices that align with market dynamics and consumer behavior.
Predictive Analytics Applications | Industries Benefiting |
---|---|
Sales Forecasting | Retail |
Disease Prediction | Healthcare |
Supply Chain Management | Manufacturing |
Customer Churn Prediction | Telecommunications |
For further exploration of AI’s role in transforming business landscapes, you can refer to the detailed insights available at Forbes and examine recent developments in this field through the latest research published by Gartner.
Unveiling the Mechanics of AI-Driven Analytics
The inner workings of AI-driven analytics hinge on the ability to sift through vast amounts of data and identify patterns that humans might overlook. By leveraging advanced algorithms, these systems can predict future trends by analyzing historical data, making them invaluable to industries such as finance, healthcare, and marketing. Key components of this analytical framework include:
- Machine Learning Models: These models adapt and improve as they process more data, enhancing their predictive accuracy over time.
- Data Visualization: By transforming complex datasets into intuitive visual formats, stakeholders can easily interpret results and make informed decisions.
- Natural Language Processing: This allows systems to understand and analyze text data, offering deeper insights into customer sentiment and market trends.
An often overlooked element is the integration of real-time data streams, which enrich AI models and provide timely insights that are crucial for decision-making. This dynamic capability allows organizations to stay ahead of their competition by responding promptly to emerging trends. As AI-driven analytics mature, innovations such as automatic feature engineering and enhanced data governance protocols will further elevate their impact. For a deeper understanding of these transformative technologies, exploring resources like Forbes on AI in Business Analytics can shed light on their practical applications and future directions.
Enhancing Decision-Making with Predictive Models
Predictive models act as a guiding compass in the ocean of data, allowing organizations to navigate complex decision-making landscapes with confidence. By analyzing historical data and identifying patterns, these models help forecast future trends and behaviors. This capability enables businesses to transform raw data into actionable insights, ultimately influencing a wide range of operational areas, from resource allocation to customer engagement. With the help of advanced algorithms, organizations can make well-informed decisions that not only address immediate concerns but also anticipate future challenges.
To illustrate the power of predictive analytics, consider the following areas where decision-making is significantly enhanced:
- Marketing Strategy: By predicting customer preferences, businesses can tailor campaigns effectively.
- Inventory Management: Anticipating demand reduces excess stock and shortages.
- Risk Assessment: Identifying potential risks early allows for proactive mitigation strategies.
The ability to predict outcomes leads to smarter, data-driven choices. A recent study by McKinsey highlights that firms leveraging predictive analytics can enhance their decision-making processes, resulting in up to a 20% increase in operational efficiency. By integrating these models into their strategic framework, organizations can not only adapt to changing market dynamics but can also thrive amidst uncertainty. For more in-depth insights, visit McKinsey’s insights on predictive analytics.
Best Practices for Implementing AI in Predictive Strategies
Effective implementation of AI in predictive strategies requires a deliberate approach that hinges on an understanding of both the technology and the domain in which it is being applied. Prior to deployment, it’s crucial to define clear objectives that align with business goals. This not only provides a framework for measuring success but also helps in choosing the right algorithms and data sources. Establishing a multidisciplinary team can facilitate communication between data scientists and domain experts, ensuring that insights generated by AI tools are contextually relevant and actionable. This collaborative effort paves the way for building models that are not only accurate but are also adaptable to changing business needs.
Furthermore, a strong emphasis on data quality and governance cannot be overstated. AI models thrive on high-quality, well-structured data, which necessitates a robust data management protocol. Regularly auditing data sources and implementing rigorous preprocessing methods are essential strategies. The following best practices can serve as a guide for organizations looking to refine their approach to AI in predictive analytics:
- Prioritize Data Integrity: Regular checks to ensure data accuracy and completeness.
- Iterate and Experiment: Continuously refine models based on feedback and new findings.
- Document Processes: Maintain thorough documentation of data sources, model decisions, and outcomes.
- Invest in Training: Equip teams with the skills needed to adapt to evolving AI technologies.
Strategy | Benefit |
---|---|
Collaborative Teams | Enhanced insights through diverse expertise. |
Continuous Learning | Models that evolve with user feedback. |
Data Governance | High-quality data leading to better predictions. |
For deeper insights into these best practices, consider exploring [McKinsey’s report on AI and analytics](https://www.mckinsey.com/capabilities/quantumblack/our-insights/ai-and-analytics) or [Gartner’s guidelines on implementing AI](https://www.gartner.com/en/information-technology/insights/artificial-intelligence). These resources provide further examples and case studies that can enrich your understanding of successful AI strategies in predictive analytics.
Concluding Remarks
As we stand on the precipice of an AI-driven future, the role of predictive analytics is becoming increasingly vital. By harnessing the power of data and advanced algorithms, businesses can gain insights that were once obscured by complexity. This transformative technology is not just about enhancing efficiency or driving profits; it’s a tool for understanding human behavior, anticipating market trends, and ultimately making informed decisions that can reshape industries.
In embracing AI for predictive analytics, organizations must also navigate the ethical landscape surrounding data use and algorithmic transparency. This journey is as much about fostering trust as it is about innovation. As we look ahead, it becomes clear that the harmony of human intuition and machine intelligence will define the next era of decision-making.
predictive analytics powered by AI offers a glimpse into a future where data-driven insights lead to more responsive, adaptive, and informed organizations. The synergy between humans and technology holds immense potential, and as this field continues to evolve, the possibilities are boundless. May we approach this frontier with curiosity, responsibility, and a shared vision for a smarter tomorrow.