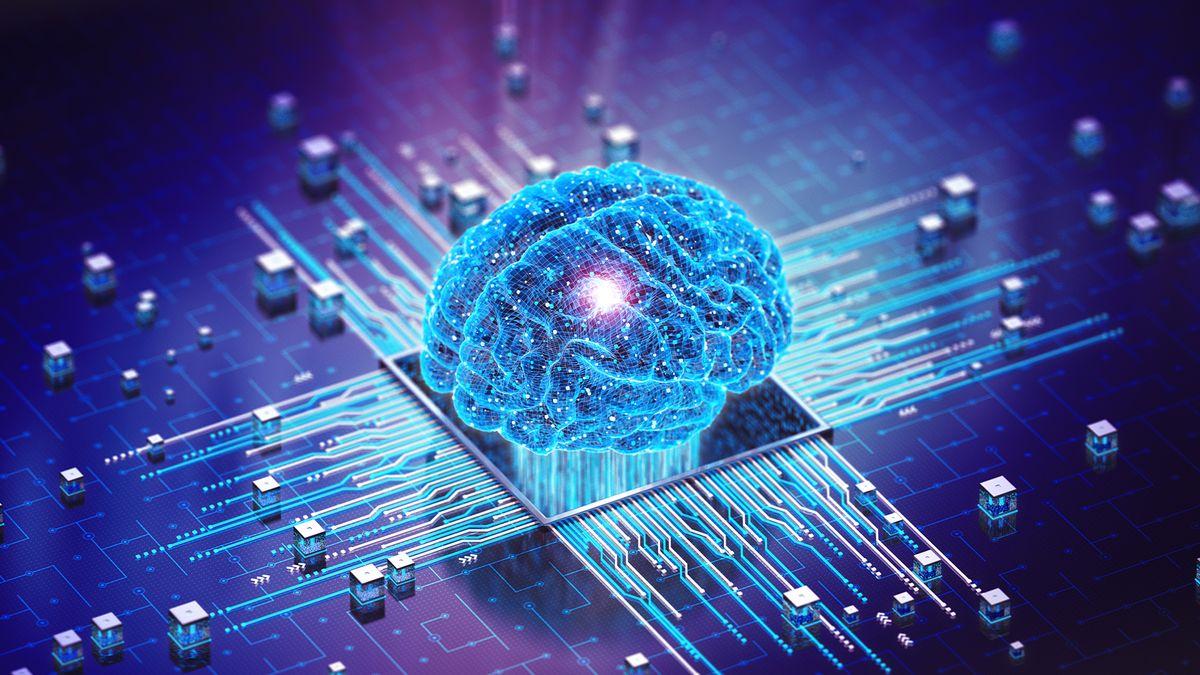
AI in machine learning
in a world increasingly driven by data, Artificial Intelligence (AI) has emerged as the lighthouse guiding us through the vast ocean of information. At the heart of this revolution lies Machine Learning (ML), a subset of AI that empowers systems to learn and adapt autonomously, transforming raw data into actionable insights.From self-driving cars to personalized recommendations on streaming services, Machine Learning is no longer a futuristic concept; it is an integral part of our daily lives, influencing decisions and shaping industries. This article delves into the intricate relationship between AI and Machine Learning, exploring the fundamental principles that underpin these technologies, their real-world applications, and the ethical considerations that accompany their deployment. Join us as we navigate the landscape of innovation, unveiling the profound impact of AI in the realm of Machine Learning and its potential to redefine the future.
Table of Contents
- Harnessing Data: The Integral Role of AI in Enhancing Machine Learning Models
- Understanding Algorithms: How AI Innovations Are Shaping predictive Analytics
- Real-World Applications: Transformative Impacts of AI on Industries Through Machine Learning
- Best Practices for Implementation: Guiding Principles to maximize AI and Machine Learning Synergy
- The Way Forward
Harnessing Data: The Integral Role of AI in enhancing Machine Learning Models
At the heart of all machine learning advancements lies the ability to harness and interpret vast amounts of data, a feat made exponentially more powerful by the integration of artificial intelligence.By deploying AI techniques like deep learning and neural networks, data can be not only processed but understood in ways previously thought unachievable. this transformative process involves intricate algorithms capable of identifying patterns, making predictions, and enhancing the model’s ability to generalize from training datasets. As these technologies mature, the relationship between AI and machine learning becomes increasingly symbiotic, with AI serving as the engine that drives machine learning’s capabilities to new heights.
The refinement of machine learning models via AI extends across several key areas, notably in automating data preprocessing, improving accuracy, and enabling real-time analytics.To illustrate the advantages visually, consider the following table that summarizes AI’s contributions to machine learning model enhancement:
Contribution | Description |
---|---|
Automated Feature Selection | AI algorithms can identify the most impactful features in large datasets, improving model efficiency. |
Adaptive Learning | Models can learn from new data trends in real-time, allowing for dynamic adjustments and enhancing predictive accuracy. |
Data augmentation | AI can generate synthetic data to increase the diversity of training datasets, mitigating overfitting. |
By employing these techniques, organizations can not only bolster the performance of their machine learning models but also reduce the time and resources needed for model progress. Consequently, the collaboration between AI and machine learning offers a pathway toward smarter, more robust applications in various fields, from healthcare to finance. The potential of this synergy illustrates why staying informed about advancements in AI is crucial for anyone looking to leverage data effectively in machine learning endeavors.For those interested in delving deeper into this topic, consider exploring [this insightful article](https://www.example.com/ai-ml-advancements) that outlines the latest trends in the integration of AI with machine learning technologies.
Understanding Algorithms: how AI Innovations Are Shaping Predictive Analytics
Algorithms are the backbone of artificial intelligence,driving complex predictions and insights in various domains. By harnessing vast amounts of data, these algorithms enable predictive analytics to transition from mere guesswork to precise forecasting. Techniques such as regression analysis, decision trees, and neural networks form the kernel of this transformation, allowing businesses to anticipate trends and consumer behaviors with remarkable accuracy.This level of foresight isn’t just theoretical; it actively shapes strategic decision-making across multiple sectors, from finance to healthcare.
As AI innovations evolve, so too does the complexity of the algorithms utilized. By integrating machine learning techniques, predictive models can adapt and improve over time, becoming increasingly tailored to specific datasets. The following table highlights some key AI algorithms commonly employed in predictive analytics:
Algorithm | Application | Benefits |
---|---|---|
Linear Regression | Economic forecasting | Simplicity, interpretability |
Random Forest | customer segmentation | High accuracy, robustness |
Neural Networks | Image and speech recognition | Capability to learn complex patterns |
Not only do these algorithms drive efficiency, but they also highlight the critical role of continuous learning in AI. By constantly refining their models, organizations can capitalize on real-time data, resulting in enhanced decision-making. For a deeper exploration of AI’s impact on predictive analytics, consider visiting [Towards Data Science](https://towardsdatascience.com/) or [Harvard Business Review](https://hbr.org/).
Real-World Applications: Transformative Impacts of AI on Industries Through Machine Learning
the impact of machine learning within various industries is profound, reshaping processes and redefining standards of efficiency. As an example, in the healthcare sector, machine learning algorithms analyze vast datasets from patient records to identify patterns that human practitioners may overlook. These technologies facilitate early diagnosis of conditions and enhance personalized medicine, tailoring treatments to individual genetics and lifestyle, ultimately improving patient outcomes.
Furthermore, the financial industry has seen transformative effects through the implementation of machine learning models for risk assessment and fraud detection.Financial institutions leverage these advanced algorithms to monitor transactions in real-time, identifying suspicious activities that deviate from established norms. This capability not only fortifies security measures but also streamlines operations, allowing institutions to allocate resources more effectively. As machine learning continues to evolve, the possibilities for its application seem limitless, paving the way for increased innovation across multiple sectors:
- Retail: Predicting customer preferences and optimizing inventory.
- Manufacturing: Enhancing predictive maintenance and supply chain optimization.
- Agriculture: Using data analytics for precision farming and crop monitoring.
Industry | Machine Learning Application | Impact |
---|---|---|
Healthcare | predictive Analytics | Improved diagnosis |
Finance | Fraud Detection | Increased security |
Retail | Customer Insights | Enhanced sales strategies |
For an in-depth understanding of how machine learning is reshaping these industries, consult Forbes. As the technology matures, staying informed about its advancements is crucial, and resources like MIT Press provide valuable insights on emerging trends.
Best Practices for Implementation: Guiding Principles to Maximize AI and Machine Learning Synergy
Fostering a robust synergy between AI and machine learning necessitates a thoughtful approach guided by established principles. Placing emphasis on data quality is crucial, as the efficacy of machine learning models largely depends on the integrity of the data fed into them. Implementing a data governance framework can safeguard against errors and inconsistencies, thus enabling cleaner datasets that streamline the learning process. Additionally, integrating continuous learning mechanisms within your AI systems allows for adaptations as new data emerges, enhancing the model’s predictive capabilities over time. This dynamic process encourages a culture where feedback loops are utilized to inform improvements, creating a self-reinforcing cycle of advancement.
Equally critically important is the necessity for interdisciplinary collaboration. Bringing together diverse skill sets from domain experts, data scientists, and IT professionals fosters a holistic view of implementation challenges. this collaborative effort can be organized through regular workshops or brainstorming sessions that leverage cross-functional insights to refine algorithms and establish shared objectives.Key factors to consider include:
- Clear Objectives: Define specific, measurable goals for AI applications.
- Scalability: Design solutions that can grow as data quantity and complexity increase.
- Ethical Guidelines: Establish a framework that ensures responsible AI practices.
For a deeper dive into these best practices, check out detailed insights from <a href="https://www.forbes.com/sites/bernardmarr/2021/05/24/the-top-5-ai-business-use-cases-in-2021/" target="blank”>forbes and explore more about <a href="https://hbr.org/2020/01/artificial-intelligence-for-the-real-world" target="blank”>Harvard business Review‘s approach to AI integration.
The Way Forward
As we conclude our exploration of AI in machine learning, it becomes clear that this dynamic duo is not just reshaping industries but redefining the very fabric of innovation. From enhancing decision-making processes to uncovering patterns within vast data landscapes,the synergy of AI and machine learning stands as a testament to human ingenuity. As we look ahead, the potential for even greater advancements looms, inviting us to imagine a future where these technologies fully realize their transformative promise.While challenges remain, the road ahead is illuminated by a blend of optimism and duty. By harnessing the power of AI thoughtfully, we can foster a brighter, more efficient future—one driven by insight, creativity, and an unwavering commitment to progress. Let us embrace this journey, fully aware that we are not just passive observers but active participants in this unfolding narrative of technological evolution.