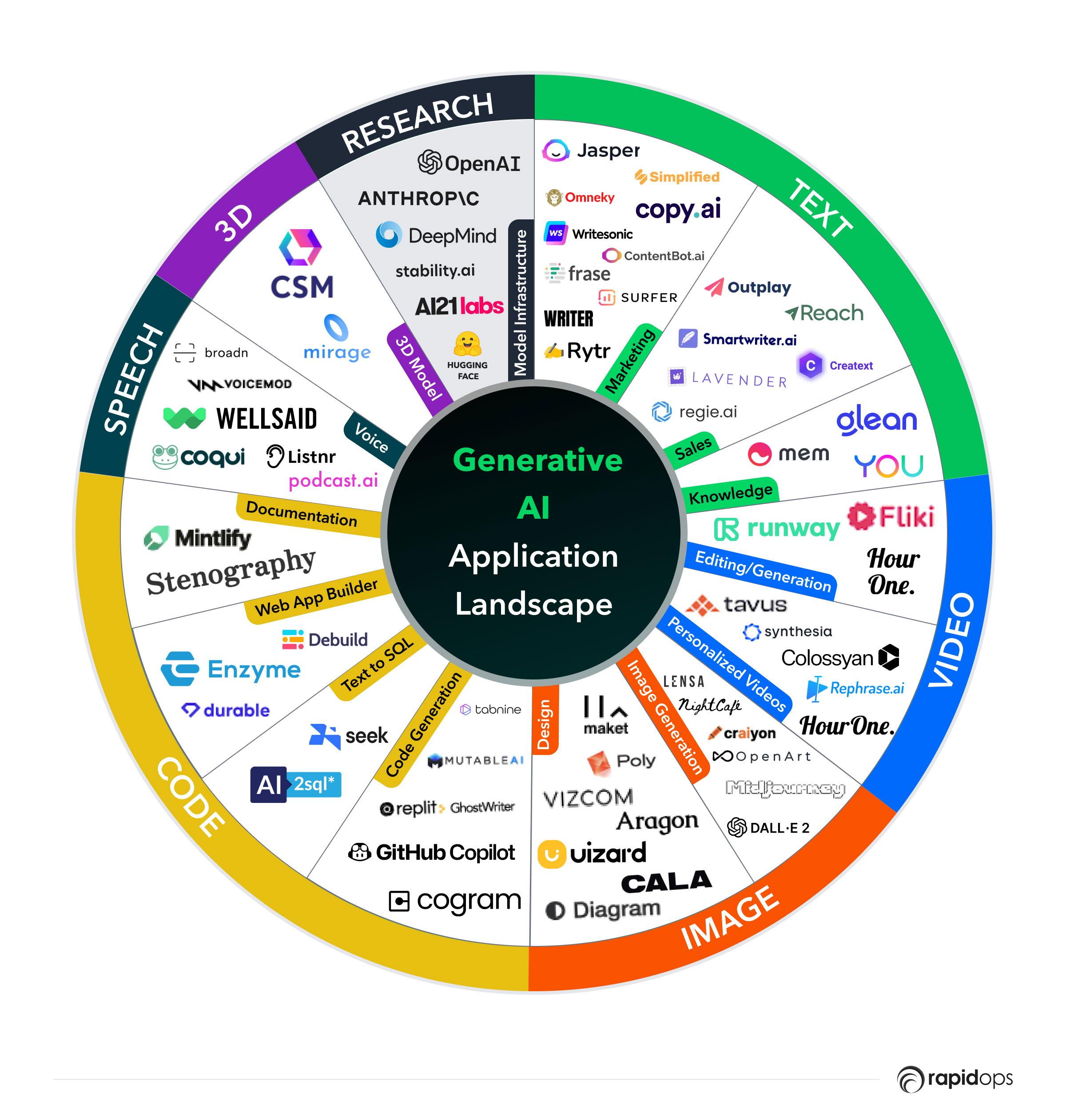
AI tools for data visualization
In today’s data-driven world, the ability too transform raw numbers into meaningful insights is more critical than ever. Enter AI tools for data visualization—a game-changing fusion of artificial intelligence and design that’s revolutionizing how we interpret complex information.Weather you’re a business leader seeking clarity in market trends, a data analyst striving for deeper insights, or a creative professional looking to tell compelling stories with data, these cutting-edge tools have somthing for everyone. In this article, we’ll explore how AI is reshaping the landscape of data visualization, highlight the most powerful tools available, and discuss ways they can empower you to unlock the full potential of your data. Ready to embrace the future of smarter, more intuitive data storytelling? Let’s dive in.
table of Contents
- Unlocking Patterns Through Machine Learning Powered Visualizations
- Choosing the Right AI Tool for Your Data Storytelling Needs
- From Raw Data to Storyboards Crafting Interactive Dashboards with AI
- Ethical Considerations When Leveraging AI in Data Visualization
- In Summary
Unlocking Patterns Through Machine Learning Powered Visualizations
Machine learning (ML) has transformed the landscape of data visualization,enabling us to reveal patterns that would or else remain invisible. By analyzing massive datasets, ML algorithms can surface correlations, trends, and anomalies that refine decision-making processes.These tools apply dimensionality reduction techniques like t-SNE or PCA, creating intuitive visual representations of complex, high-dimensional data. For example, t-SNE can project multivariable datasets into a simple two-dimensional space, making clusters stand out clearly while minimizing distortion. Such techniques are particularly vital in domains like genomics, where layered complexities often render conventional visualization methods insufficient.
What truly sets ML-powered visualizations apart is the ability to adapt and learn from user interactions. Rather than static charts, modern platforms employ interactive dashboards driven by reinforcement learning. This dynamic approach allows users to refine their queries, explore outliers, or even simulate “what-if” scenarios.Tools like Facets from Google provide an excellent starting point for exposing intricate data structures within broader datasets. The integration of adaptive models with visualization suites creates a seamless feedback loop,ensuring that insights become more actionable over time.
Choosing the right AI Tool for Your Data storytelling Needs
Crafting engaging and impactful data visualizations begins with selecting the appropriate AI tool that aligns with your goals. The landscape of AI-powered visualization tools is vast, each catering to specific needs such as ease of use, customization, or integration capabilities. To make a selection that resonates with your objectives, consider factors like the complexity of your dataset, the level of interaction required in your visuals, and the narrative you wish to convey to your audience.
Here are a few criteria to help streamline your decision-making process:
- Data Complexity: For massive datasets, tools like Tableau or power BI excel with their refined integration capabilities.
- Customization: If branding or bespoke visualization styles are vital, tools like Flourish or D3.js might be better alternatives.
- Automation: Platforms like DataRobot can simplify tasks with predictive analytics while maintaining attention-grabbing visuals.
need more guidance? Check out viz factors breakdown..</p.implement better struture eg ?
From Raw Data to Storyboards Crafting Interactive Dashboards with AI
From Raw Data to Storyboards: Crafting Interactive Dashboards with AI
Transforming raw data into insightful, interactive dashboards is an art, and with AI, it’s becoming more like storyboarding than traditional data analysis. By integrating natural language processing and machine learning, AI tools can now identify patterns within datasets that might otherwise remain hidden. They contextualize these findings into visually rich dashboards, translating abstract numbers into narratives that users can immediately grasp. This capability bridges the gap between data professionals and stakeholders, ensuring decisions are based on clarity rather than complexity.
Some AI-powered tools even allow users to bypass coding entirely by using drag-and-drop interfaces or by interpreting commands in plain language. For example, tools like Tableau (when combined with AI extensions) or Microsoft Power BI’s AI integrations make it possible to iterate quickly and refine designs to tell the most compelling story. Here’s a rapid comparison of two approaches:
Feature | AI-Powered Dashboards | traditional Tools |
---|---|---|
User Accessibility | Beginner-pleasant; no coding required | Advanced knowledge frequently enough needed |
Speed | Automates data prep & analysis | Manual data manipulation takes longer |
For those ready to explore, platforms like <a href="https://www.tableau.com" target="blank” rel=”nofollow”>Tableau or emerging AI-driven analytics from <a href="https://powerbi.microsoft.com" target="blank” rel=”nofollow”>Power BI can elevate your storytelling process.The future of dashboards is less about generating static reports and more about crafting dynamic, evolving storyboards that respond to the questions users didn’t even know they had.
Ethical Considerations When Leveraging AI in Data Visualization
Integrating AI into data visualization introduces a powerful set of tools, but it also raises critical ethical concerns that cannot be ignored. One key issue is algorithmic bias, where AI systems may unintentionally reflect or amplify biases present in the data. For example, if datasets lack diversity or contain past inequities, AI-driven visualizations might misrepresent trends, leading to flawed insights. This is particularly problematic in fields like healthcare or public policy,where decisions based on biased visuals can have far-reaching consequences. To mitigate this, it’s essential to conduct thorough audits of training data and employ transparent AI methodologies. Organizations like the partnership on AI offer guidance to promote ethics and fairness in AI applications.
Another consideration is the potential misuse of AI-powered visualizations.With the advanced capabilities to generate visually compelling outputs, there is a risk of creating deceptive or misleading graphics. for instance, skewed axis scales or selective data filtering may exaggerate trends to sway public opinion or support biased narratives. Professionals must adopt responsible data storytelling practices, ensuring clarity, proportionality, and honest representation of information. A practical measure is employing peer reviews within teams to cross-check visual accuracy and integrity before publishing. For those looking to explore these principles further, our recent post on ethical data storytelling with AI dives deeper into maintaining trust when designing visuals.
In Summary
In the ever-evolving landscape of data visualization,AI tools have emerged as powerful allies,transforming the way we interpret,interact with,and communicate complex information. From streamlining workflows to uncovering hidden patterns, these technologies are more than just tools—they’re partners in discovery. However, as we integrate AI into our visualization processes, it’s essential to remain thoughtful, balancing automation with human intuition to create meaningful, impactful narratives.The canvas of data is expansive, and with the right blend of creativity and technology, the possibilities are as limitless as the datasets themselves. so, as you explore this dynamic intersection of AI and visualization, remember: the story behind the numbers is waiting for you to bring it to light.